Eric Herzog, CMO at Infinite
Generative Artificial Intelligence (GenAI) found an unexpected “partner” in an IT field that CIOs don’t typically prioritize when it comes to artificial intelligence – corporate magazine. Because data is critical to activating and controlling GenAI, the storage infrastructure that stores all enterprise data has taken on a modern role as the basis for recovery-assisted generation (RAG).
RAG is very useful for any enterprise that plans to utilize GenAI for personalized query responses. RAG is a GenAI-centric platform for extending, refining, and optimizing the output of artificial intelligence models such as enormous language models (LLM) and diminutive language models (SLM).
Here’s what you need to know: RAG is a storage infrastructure-based architecture that improves AI accuracy. It enables enterprises to ensure that responses from AI models remain relevant, timely and in the right context. With the powerful capabilities of generative AI, AI models support smart chatbots and other natural language processing applications that are used to answer user questions by referencing credible sources of information.
Many AI models are initially trained on extremely enormous datasets that are typically publicly available. However, to ensure that responses to customer questions are highly detailed and contextually correct for your enterprise, RAG redirects the artificial intelligence model (i.e. LLM) to pull private and proprietary data from your organization’s databases. This is key to improving AI accuracy because it leverages reliable, predetermined, internal sources of knowledge – all without requiring resource-intensive retraining of the AI model.
CIOs and business leaders overseeing GenAI projects can breathe a sigh of relief. With this modern option to extend the usability of enterprise storage infrastructure to improve AI accuracy, enterprises can now cost-effectively add an information retrieval component to GenAI deployments and rely on their internal data sets to avoid exposing their enterprise to public inaccuracies. As part of the transformation effort to lead the company into an AI-enhanced future, this is an opportunity to leverage smart automation with RAG to create better, more exact and faster responses.
No specialized equipment needed
Part of the good news about the RAG workflow deployment architecture is that it doesn’t require any specialized hardware. Existing enterprise storage systems such as InfiniBox® and InfiniBox™ SSA can be leveraged to implement RAG to achieve this added value component of process improvement and improvement, making GenAI more exact and relevant.
RAG adds a whole modern dimension to the business value of enterprise storage to augment GenAI success rates in enterprise-sized organizations. This involves leveraging enterprise storage for CIOs to utilize when building an ecosystem of an AI model optimized with RAG. It becomes a necessity.
To take full advantage of RAG, you need the highest performance of your storage array and 100% availability guaranteed by an SLA. Never before has 100% enterprise storage availability been as critical to business operations as it is today in a GenAI-powered world. It is also prudent to add cyber resiliency capabilities to your data infrastructure to ensure cyber data recovery, which is an integral part of GenAI applications.
Whether all data resides in a data center or in a hybrid multi-cloud setup, the RAG workflow deployment architecture will work. The cloud version of the enterprise storage solution integrates seamlessly with the cloud, simplifying and accelerating RAG deployment across enterprises. This complements the work that hyperscalers are doing to build larger-scale AI models for initial AI model training.
Why is RAG so critical to GenAI?
Even if the initial training phase goes exceptionally well, AI models still pose challenges for enterprises. Too often, they can cause “AI hallucinations,” which are essentially wrong or misleading GenAI model outputs. If it doesn’t have the information it needs, the AI model will come up with an answer just to get the answer, even if that answer is based on false information. This eroded the trust people had in initial GenAI implementations.
AI models tend to provide wrong answers due to confusion in terminology. They may also provide old-fashioned information or an answer from an unreliable source. The consequence of this is that the company’s customer may obtain completely wrong information without even knowing it. What a “data disaster” this is!
RAG addresses this set of challenges head-on. This is a reliable method to eliminate “AI hallucinations” and provide more informed query responses through GenAI enterprise applications. The AI learning model leverages modern knowledge from the RAG workflow, as well as training data, to create significantly better responses. This will improve the level of trust people have in GenAI.
Key takeaways
Thanks to the RAG architecture, enterprise storage is now an imperative element of GenAI implementation strategies. Employ it to continuously refine your RAG pipeline with modern, up-to-date data to improve the accuracy of your AI models.
Be sure not to underutilize your own proprietary data sets stored in your company’s databases. You need to connect the dots between GenAI and data infrastructure. RAG’s enterprise storage-based solution will aid you.
To optimize your storage systems for this improvement, look for industry-leading performance, 100% availability and cyber resiliency of storage. Thanks to them, you will be ready for RAG.
Metaphorically, RAG is like “new oil” that makes the GenAI engine perform better with trusted data in an always-on data infrastructure.
About Eric Herzog
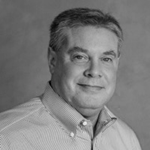
Eric Herzog is the company’s chief marketing officer Infinite. Prior to joining Infinidat, Herzog was CMO and Vice President of Global Storage Channels at IBM Storage Solutions. His leadership experience also includes: Director of Marketing and Senior Vice President of Alliances at all-flash storage provider Violin Memory and Senior Vice President of Product Management and Product Marketing for EMC’s Enterprise and Midrange Systems Division.